CS 7476 Advanced Computer Vision
Fall 2020, MW 2:00 to 3:15, Van Leer C341 (but mostly online)
Instructor: James Hays
TA: Sean Foley
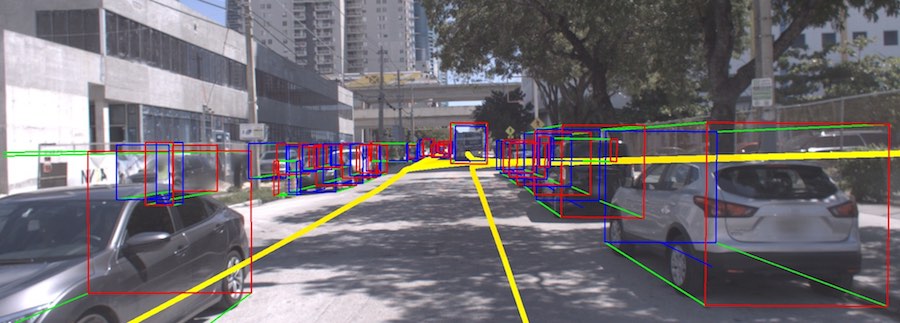
Course Description
This course covers advanced research topics in computer vision. Building on the introductory materials in CS 6476 (Computer Vision), this class will prepare graduate students in both the theoretical foundations of computer vision as well as the practical approaches to building real Computer Vision systems. This course investigates current research topics in computer vision with an emphasis on recognition tasks and deep learning. We will examine data sources, features, and learning algorithms useful for understanding and manipulating visual data. This year, special emphasis will be placed on research surrounding autonomous vehicles. Class topics will be pursued through independent reading, class discussion and presentations, and state-of-the-art projects.The goal of this course is to give students the background and skills necessary to perform research in computer vision and its application domains such as robotics, healthcare, and graphics. Students should understand the strengths and weaknesses of current approaches to research problems and identify interesting open questions and future research directions. Students will hopefully improve their critical reading and communication skills, as well.
Course Requirements
Reading and Discussion Topics
Students will be expected to read one paper for each class. For each assigned paper, students must identify at least one question or topic of interest for class discussion. Interesting topics for discussion could relate to strengths and weaknesses of the paper, possible future directions, connections to other research, uncertainty about the conclusions of the experiments, etc. Questions / Discussion topics must be posted to the course Canvas discussions tab by 11:59pm the day before each class. Feel free to reply to other comments and help each other understanding confusing aspects of the papers. The Canvas discussion will be the starting point for the class discussion. If you are presenting you don't need to post a question to Canvas.Class participation
All students are expected to take part in class discussions. If you do not fully understand a paper that is OK. We can work through the unclear aspects of a paper together in class. If you are unable to attend a specific class please let me know ahead of time (and have a good excuse!).This fall, lectures will be virtual through Bluejeans (find the link in Canvas). But the expectations for participation remain. Discussion questions can be asked through text rather than voice, if preferred.
Presentation(s)
Each student will lead the presentation of one paper during the semester (possibly as part of a pair of students). Ideally, students would implement some aspect of the presented material and perform experiments that help us to understand the algorithms. Presentations and all supplemental material should be ready one week before the presentation date so that students can meet with the instructor, go over the presentation, and possibly iterate before the in-class discussion. For the presentations it is fine to use slides and code from outside sources (for example, the paper authors) but be sure to give credit.Semester group projects
Students will work alone or in pairs to complete a state-of-the-art research project on a topic relevant to the course. Students will propose a research topic early in the semester. After a project topic is finalized, students will meet occasionally with the instructor or TA to discuss progress. Students will report their progress on their semester project twice during the course and the course will end with final project presentations. Students will also produce a conference-formatted write-up of their project. Projects will be published on the this web page. The ideal project is something with a clear enough direction to be completed in a couple of months, and enough novelty such that it could be published in a peer-reviewed venue with some refinement and extension.Prerequisites
Strong mathematical skills (linear algebra, calculus, probability and statistics) are needed. It is strongly recommended that students have taken one of the following courses (or equivalent courses at other institutions):- Computer Vision CS 4476 / 6476
- Machine Learning
- Deep Learning
- Computer Graphics
- Computational Photography
Textbook
We will not rely on a textbook, although the free, online textbook "Computer Vision: Algorithms and Applications, 2nd edition" by Richard Szeliski is a helpful resource.Grading
Your final grade will be made up from- 20% Reading summaries and questions posted to Canvas
- 10% Classroom participation and attendance
- 15% Leading discussion for particular research paper
- 20% Semester project updates
- 35% Semester project
Office Hours:
James Hays, Wednesdays after lectureSean Foley, Mondays after lecture
Tentative Schedule
Date | Paper | Paper, Project page | Presenter |
Mon, Aug 17 | Course overview | James | |
Wed, Aug 19 | Review of driving research tasks | James | |
Mon, Aug 24 | Review of 2D object detection | Sean | |
Wed, Aug 26 | Driving datasets, semester project proposal process | James | |
Mon, Aug 31 | Stanley: The Robot that Won the DARPA Grand Challenge | Patrick | |
Wed, Sep 2 | PointNet: Deep Learning on Point Sets for 3D Classification and Segmentation. Charles R. Qi, Hao Su, Kaichun Mo, Leonidas J. Guibas. 2017 | arXiv | Nitish |
Mon, Sep 7 | No Classes, Institute Holiday | ||
Wed, Sep 9 | Fine-grained Recognition in the Wild: A Multi-Task Domain Adaptation Approach. Timnit Gebru, Judy Hoffman, Li Fei-Fei. 2017 | arXiv | |
Mon, Sep 14 | VoxelNet: End-to-End Learning for Point Cloud Based 3D Object Detection. Yin Zhou, Oncel Tuzel. 2017 | arXiv | |
Wed, Sep 16 | HDNET: Exploiting HD Maps for 3D Object Detection. Bin Yang, Ming Liang, Raquel Urtasun. 2018 | ||
Mon, Sep 21 | PointPillars: Fast Encoders for Object Detection from Point Clouds. Alex H. Lang, Sourabh Vora, Holger Caesar, Lubing Zhou, Jiong Yang, Oscar Beijbom. 2018 | arXiv | |
Wed, Sep 23 | Pseudo-LiDAR from Visual Depth Estimation: Bridging the Gap in 3D Object Detection for Autonomous Driving. Yan Wang, Wei-Lun Chao, Divyansh Garg, Bharath Hariharan, Mark Campbell, Kilian Q. Weinberger. 2018 | arXiv | |
Mon, Sep 28 | Deep Hough Voting for 3D Object Detection in Point Clouds. Charles R. Qi, Or Litany, Kaiming He, Leonidas J. Guibas. 2019 | arXiv | |
Wed, Sep 30 | SinGAN: Learning a Generative Model from a Single Natural Image. Tamar Rott Shaham, Tali Dekel, Tomer Michaeli. 2019 | arXiv | |
Mon, Oct 5 | Rules of the Road: Predicting Driving Behavior with a Convolutional Model of Semantic Interactions. Joey Hong, Benjamin Sapp, James Philbin. 2019 | arXiv | |
Wed, Oct 7 | Towards Robust Monocular Depth Estimation: Mixing Datasets for Zero-shot Cross-dataset Transfer. Rene Ranftl, Katrin Lasinger, David Hafner, Konrad Schindler, Vladlen Koltun. 2019 | arXiv | |
Mon, Oct 12 | Towards Fairness in Visual Recognition: Effective Strategies for Bias Mitigation. Zeyu Wang, Klint Qinami, Ioannis Christos Karakozis, Kyle Genova, Prem Nair, Kenji Hata, Olga Russakovsky. 2019 | arXiv | |
Wed, Oct 14 | SuperGlue: Learning Feature Matching with Graph Neural Networks. Paul-Edouard Sarlin, Daniel DeTone, Tomasz Malisiewicz, Andrew Rabinovich. 2019 | arXiv | |
Mon, Oct 19 | Password-conditioned Anonymization and Deanonymization with Face Identity Transformers. Xiuye Gu, Weixin Luo, Michael S. Ryoo, Yong Jae Lee. 2019 | arXiv | |
Wed, Oct 21 | End-to-End Model-Free Reinforcement Learning for Urban Driving using Implicit Affordances. Marin Toromanoff, Emilie Wirbel, Fabien Moutarde. 2019 | arXiv | |
Wed, Oct 21 | Learning a Neural Solver for Multiple Object Tracking. Guillem Braso, Laura Leal-Taixe. 2019 | arXiv | |
Mon, Oct 26 | BBN: Bilateral-Branch Network with Cumulative Learning for Long-Tailed Visual Recognition. Boyan Zhou, Quan Cui, Xiu-Shen Wei, Zhao-Min Chen. 2019 | arXiv | |
Wed, Oct 28 | Predicting Semantic Map Representations from Images using Pyramid Occupancy Networks. Thomas Roddick, Roberto Cipolla. 2020 | arXiv | |
Mon, Nov 2 | NeRF: Representing Scenes as Neural Radiance Fields for View Synthesis. Ben Mildenhall, Pratul P. Srinivasan, Matthew Tancik, Jonathan T. Barron, Ravi Ramamoorthi, Ren Ng. 2020 | arXiv | |
Wed, Nov 4 | PolarNet: An Improved Grid Representation for Online LiDAR Point Clouds Semantic Segmentation. Yang Zhang, Zixiang Zhou, Philip David, Xiangyu Yue, Zerong Xi, Boqing Gong, Hassan Foroosh. 2020 | arXiv | |
Mon, Nov 9 | STINet: Spatio-Temporal-Interactive Network for Pedestrian Detection and Trajectory Prediction. Zhishuai Zhang, Jiyang Gao, Junhua Mao, Yukai Liu, Dragomir Anguelov, Congcong Li. 2020 | arXiv | |
Wed, Nov 11 | SurfelGAN: Synthesizing Realistic Sensor Data for Autonomous Driving. Zhenpei Yang, Yuning Chai, Dragomir Anguelov, Yin Zhou, Pei Sun, Dumitru Erhan, Sean Rafferty, Henrik Kretzschmar. 2020 | arXiv | |
Wed, Nov 11 | End-to-End Object Detection with Transformers. Nicolas Carion, Francisco Massa, Gabriel Synnaeve, Nicolas Usunier, Alexander Kirillov, Sergey Zagoruyko. 2020 | arXiv | |
Mon, Nov 16 | Towards Streaming Perception. Mengtian Li, Yu-Xiong Wang, Deva Ramanan. 2020 | arXiv | |
Mon, Nov 16 | PnPNet: End-to-End Perception and Prediction with Tracking in the Loop. Ming Liang, Bin Yang, Wenyuan Zeng, Yun Chen, Rui Hu, Sergio Casas, Raquel Urtasun. 2020 | arXiv | |
Wed, Nov 18 | What Matters in Unsupervised Optical Flow. Rico Jonschkowski, Austin Stone, Jonathan T. Barron, Ariel Gordon, Kurt Konolige, Anelia Angelova. 2020 | arXiv | |
Wed, Nov 18 | Pruning neural networks without any data by iteratively conserving synaptic flow. Hidenori Tanaka, Daniel Kunin, Daniel L. K. Yamins, Surya Ganguli. 2020 | arXiv | |
Mon, Nov 23 | Contrastive Learning for Unpaired Image-to-Image Translation. Taesung Park, Alexei A. Efros, Richard Zhang, Jun-Yan Zhu. 2020 | arXiv | |
Mon, Nov 23 | TNT: Target-driveN Trajectory Prediction. Hang Zhao, Jiyang Gao, Tian Lan, Chen Sun, Benjamin Sapp, Balakrishnan Varadarajan, Yue Shen, Yi Shen, Yuning Chai, Cordelia Schmid, Congcong Li, Dragomir Anguelov. 2020 | arXiv | |
Final Exam Slot Monday, Dec 7, 2:40 to 5:30 |
Final Project Presentations | Everyone | |
Thursday, Dec 10 | Final Report due | Everyone |