Quality Assessment for Crownsourced Object Annotations
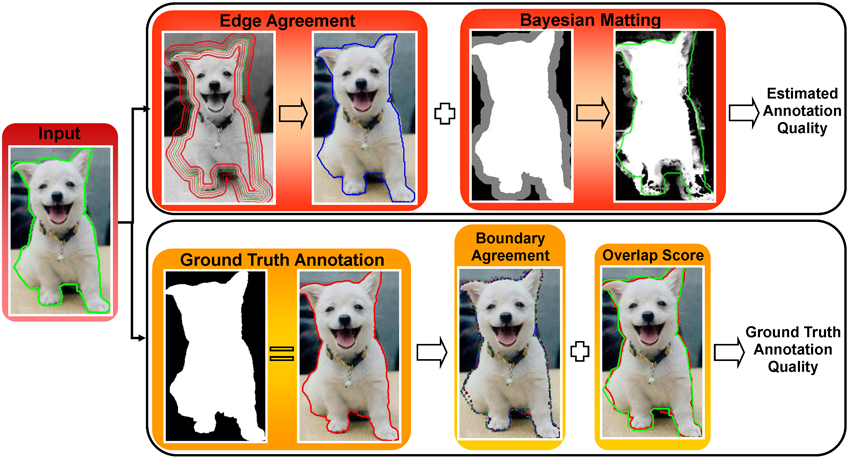
People
Abstract
As computer vision datasets grow larger the community is increasingly relying on crowdsourced annotations to train and test our algorithms. Due to the heterogeneous and unpredictable capability of online annotators, various strategies have been proposed to "clean" crowdsourced annotations. However, these strategies typically involve getting more annotations, perhaps different types of annotations (e.g. a grading task), rather than computationally assessing the annotation or image content. In this paper we propose and evaluate several strategies for automatically estimating the quality of a spatial object annotation. We show that one can significantly outperform simple baselines, such as that used by LabelMe, by combining multiple image-based annotation assessment strategies.
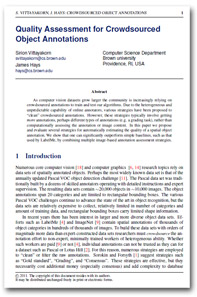
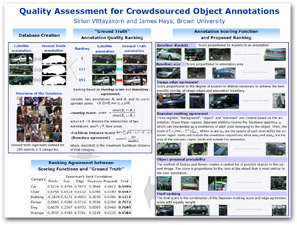
Paper
Sirion Vittayakorn, James Hays. Quality Assessment for Crownsourced Object Annotations. Proceeding of British Machine Vision Conference (BMVC), 2011.
paper pdf (11 MB),
Bibtex
Poster
poster pdf (1 MB)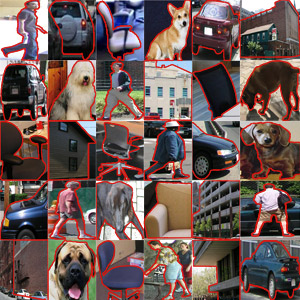
Database of LabelMe and Ground Truth Annotations
This database contains 1000 image crops -- 200 each from 5 object categories. For each crop we provide an accurate "ground truth" annotation generated according to LabelMe guidelines.