Yunan Luo
Assistant Professor
Computational Science and Engineering
Georgia Institute of Technology
Email: yunan [at] gatech.edu
Office: CODA S1245
Address: 756 W Peachtree St NW, Atlanta, GA 30308
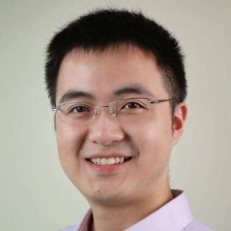
I am an Assistant Professor in the School of Computational Science and Engineering (CSE), Georgia Institute of Technology since January 2022. I received my PhD in 2021 from the Department of Computer Science at the University of Illinois Urbana-Champaign, advised by Prof. Jian Peng. Prior to that, I received my bachelor’s degree in Computer Science from Yao Class at Tsinghua University in 2016.
I am broadly interested in computational biology and machine learning, with a focus on developing AI and data science methods to reveal core scientific insights into biology and medicine. Recent interests include machine learning, geometric deep learning, generative models, network and system biology, functional genomics, cancer genomics, drug repositioning and discovery, and AI-guided protein engineering.
Recent News
- [2023/12] Our paper on contrastive fitness learning accpeted by RECOMB 2024.
- [2023/11] KDBNet paper published in Nature Machine Intelligence.
- [2023/09] Received an Azure grant from Microsoft’s Accelerating Foundation Models Research Initiative.
- [2023/09] We received the NIH MIRA award!
- [2023/09] We received the Seed Grant Award from the NSF AI Institute MMLI.
- [2023/04] We received the Amazon Research Award.
- [2023/04] One paper on network alignment accepted to ISMB 2023.
- [2023/03] Our enzyme function prediction paper published at Science!
Selected Awards
- NIH Maximizing Investigators’ Research Award (MIRA) (R35 for ESI), 2023
- Top-10 Reviewer Award, LOG Conference, 2023
- Amazon Research Award, 2022
- Top-20 Reviewer Award, LOG Conference, 2022
Teaching
- CSE7850/CX4803, Machine Learning for Computational Biology, Spring 2024
- CSE8803 MLG, Machine Learning with Graphs, Fall 2023
- CSE8803/CX4803, Machine Learning for Computational Biology, Spring 2023
- CSE8803/CX4803, Machine Learning for Computational Biology, Spring 2022 (with Xiuwei Zhang)
Papers
[Google Scholar](*=equal contribution)
- Contrastive Fitness Learning: Reprogramming Protein Language Models for Low-N Learning of Protein Fitness LandscapeRECOMB, 2024 (accepted)
- Calibrated geometric deep learning improves kinase-drug binding predictionsNature Machine Intelligence, 2023
- LinkerNet: Fragment Poses and Linker Co-Design with 3D Equivariant DiffusionNeurIPS, 2023
- Supervised biological network alignment with graph neural networksISMB, 2023
- Metabolic activity organizes olfactory representationseLife, 2023
- Enzyme function prediction using contrastive learningScience, 2023
- Sensing the shape of functional proteins with topologyNature Computational Science, 2023 (News & Views)
- Interpretable Geometric Deep Learning via Learnable Randomness InjectionICLR, 2023
- Contrastive learning of protein representations with graph neural networks for structural and functional annotationsPSB, 2023
- Next Decade’s AI-Based Drug Development Features Tight Integration of Data and ComputationHealth Data Science, 2022 (Perspective)
- scPretrain: multi-task self-supervised learning for cell-type classificationBioinformatics, 2022
- ECNet is an evolutionary context-integrated deep learning framework for protein engineeringNature Communications, 2021
- Deep geometric representations for modeling effects of mutations on protein-protein binding affinityPLoS computational biology, 2021
- Few-shot learning creates predictive models of drug response that translate from high-throughput screens to individual patientsNature Cancer, 2021
- Crowdsourced mapping of unexplored target space of kinase inhibitorsNature Communications, 2021
- An integrative drug repositioning framework discovered a potential therapeutic agent targeting COVID-19Signal Transduction and Targeted Therapy, 2021
- Evolutionary context-integrated deep sequence modeling for protein engineeringRECOMB, 2020
- Characterization of SARS-CoV-2 viral diversity within and across hostsbioRxiv:2020.05.07.083410, 2020
- STAIR 2.0: A Generic and Automatic Algorithm to Fuse Modis, Landsat, and Sentinel-2 to Generate 10 m, Daily, and Cloud-/Gap-Free Surface Reflectance ProductRemote Sensing, 2020
- Deriving high-spatiotemporal-resolution leaf area index for agroecosystems in the U.S. Corn Belt using Planet Labs CubeSat and STAIR fusion dataRemote Sensing of Environment, 2020
- Integrating Deep Neural Networks and Symbolic Inference for Organic Reactivity PredictionchemRxiv:11659563, 2020
- Sequence Modeling of Temporal Credit Assignment for Episodic Reinforcement LearningarXiv:1905.13420, 2019
- DeepMask: An algorithm for cloud and cloud shadow detection in optical satellite remote sensing images using deep residual networkarXiv:1911.03607, 2019
- Integrating thermodynamic and sequence contexts improves protein-RNA binding predictionPLoS computational biology, 2019 (Presented at GLBIO’19)
- Mitigating Data Scarcity in Protein Binding Prediction Using Meta-LearningRECOMB, 2019
- Metagenomic binning through low-density hashingBioinformatics, 2018
- STAIR: A generic and fully-automated method to fuse multiple sources of optical satellite data to generate a high-resolution, daily and cloud-/gap-free surface reflectance productRemote Sensing of Environment, 2018
- Deciphering Signaling Specificity with Deep Neural NetworksRECOMB, 2018
- Large-scale integration of heterogeneous pharmacogenomic data for identifying drug mechanism of actionPSB, 2018
- A network integration approach for drug-target interaction prediction and computational drug repositioning from heterogeneous informationNature communications, 2017 (Extended version of the RECOMB’17 paper)
- A network integration approach for drug-target interaction prediction and computational drug repositioning from heterogeneous informationRECOMB, 2017
- Low-density locality-sensitive hashing boosts metagenomic binningRECOMB, 2016