
JACOB ABERNETHY
prof_AT_gatech_DOT_edu
2134 Klaus Advanced Computing
Georgia Institute of Technology
226 Ferst Drive NW
Atlanta, GA 30332
About Me
I am an Assistant Professor in the School of Computer Science in the College of Computing at the Georgia Institute of Technology.
My research focus is Machine Learning, and I like discovering connections between Optimization, Statistics, and Economics. I finished my PhD at UC Berkeley with Peter Bartlett in 2011, and I was a Simons postdoctoral fellow with Michael Kearns for the following two years.
Recent News
- ⇒ Thanks to the National Science Foundation for IIS Award 1910077 titled “RI: Small: Training Modularized Learning Systems Through Non-Convex Multiplayer Game Dynamics”
- ⇒ I am spending the summer of 2019 at Google Research NYC
- ⇒ Along with Shivani Agarwal, I will be the program co-chair of the 2020 Conference on Learning Theory (COLT)
- ⇒ In the years since Flint Michigan’s water crisis, I continue to work on the problem of detecting lead contamination and infrastructure remediation. More details on this project can be found here.
Current group
PhD Student
PhD Student
PhD Student
PhD Student
PhD Student
Postdoctoral Fellow
PhD Student
Former students and postdocs
Former PhD student, now works at Apple
Received PhD in 2018, now at Google
former PhD Student, currently completing PhD at MIT
former Postdoctoral Fellow, currently Assistant Professor at the University of Illinois at Urbana-Champagne
Graduated! Former PhD student in CSE. Currently a faculty member at Swarthmore College
Journal Papers
- Abernethy, J., Lai, K. A., & Wibisono, A. (2019). Last-iterate convergence rates for min-max optimization. ArXiv Preprint ArXiv:1906.02027.
- Abernethy, J., Lai, K. A., & Wibisono, A. (2019). Last-iterate convergence rates for min-max optimization. ArXiv Preprint ArXiv:1906.02027.
- Misra, K., Schwartz, E. M., & Abernethy, J. (2018). Dynamic online pricing with incomplete information using multi-armed bandit experiments. Marketing Science (to Appear).
- Abernethy, J., Lai, K. A., Levy, K. Y., & Wang, J.-K. (2018). Faster rates for convex-concave games. ArXiv Preprint ArXiv:1805.06792.
- Chojnacki, A., Dai, C., Farahi, A., Shi, G., Webb, J., Zhang, D. T., … Schwartz, E. (2017). A Data Science Approach to Understanding Residential Water Contamination in Flint. ArXiv Preprint ArXiv:1707.01591.
- Kodali, N., Abernethy, J., Hays, J., & Kira, Z. (2017). On convergence and stability of gans. ArXiv Preprint ArXiv:1705.07215.
- Abernethy, J. D., Lee, C., McMillan, A., & Tewari, A. (2017). Online Learning via Differential Privacy. CoRR, abs/1711.10019. Retrieved from http://arxiv.org/abs/1711.10019
- Abernethy, J., Anderson, C., Chojnacki, A., Dai, C., Dryden, J., Schwartz, E., … others. (2016). Data Science in Service of Performing Arts: Applying Machine Learning to Predicting Audience Preferences. ArXiv Preprint ArXiv:1611.05788.
- Abernethy, J., Lee, C., & Tewari, A. (2016). Perturbation techniques in online learning and optimization. Perturbations, Optimization, and Statistics, Neural Information Processing Series.
- Abernethy, J. D., Frongillo, R. M., & Kutty, S. (2015). On risk measures, market making, and exponential families. ACM SIGecom Exchanges, 13(2), 21–25.
- Abernethy, J., Chen, Y., & Vaughan, J. W. (2013). Efficient market making via convex optimization, and a connection to online learning. ACM Transactions on Economics and Computation, 1(2), 12.
- Abernethy, J. D., Hazan, E., & Rakhlin, A. (2012). Interior-point methods for full-information and bandit online learning. IEEE Transactions on Information Theory, 58(7), 4164–4175.
- Abernethy, J. D. (2011). Sequential Decision Making in Non-stochastic Environments.
- Abernethy, J., Chapelle, O., & Castillo, C. (2010). Graph regularization methods for web spam detection. Machine Learning Journal, 81(2), 207–225.
- Abernethy, J., Bach, F., Evgeniou, T., & Vert, J.-P. (2009). A New Approach to Collaborative Filtering: Operator Estimation with Spectral Regularization. The Journal of Machine Learning Research, 10, 803–826.
- Abernethy, J., Evgeniou, T., Toubia, O., & Vert, J.-P. (2008). Eliciting consumer preferences using robust adaptive choice questionnaires. IEEE Transactions on Knowledge and Data Engineering, 20(2), 145–155.
Conference and Workshop Papers
- Cardoso, A. R., Abernethy, J., Wang, H., & Xu, H. (2019). Competing Against Nash Equilibria in Adversarially Changing Zero-Sum Games. In K. Chaudhuri & R. Salakhutdinov (Eds.), Proceedings of the 36th International Conference on Machine Learning (Vol. 97, pp. 921–930). Long Beach, California, USA: PMLR. Retrieved from http://proceedings.mlr.press/v97/cardoso19a.html
- Wang, J.-K., & Abernethy, J. D. (2018). Acceleration through Optimistic No-Regret Dynamics. In Advances in Neural Information Processing Systems (pp. 3828–3838).
- Abernethy, J., Lai, K. A., Levy, K. Y., & Wang, J.-K. (2018). Faster Rates for Convex-Concave Games. In (COLT) Conference On Learning Theory (pp. 1595–1625).
- Abernethy, J., Chojnacki, A., Farahi, A., Schwartz, E., & Webb, J. (2018). ActiveRemediation: The Search for Lead Pipes in Flint, Michigan. In Proceedings of the 24th ACM SIGKDD International Conference on Knowledge Discovery & Data Mining (pp. 5–14). ACM. PDF** KDD 2018 Best Student Paper Award (Research Track) **
- Abernethy, J. D., & Wang, J.-K. (2017). On frank-wolfe and equilibrium computation. In Advances in Neural Information Processing Systems (pp. 6584–6593).
- Chojnacki, A., Dai, C., Farahi, A., Shi, G., Webb, J., Zhang, D. T., … Schwartz, E. (2017). A Data Science Approach to Understanding Residential Water Contamination in Flint. In Proceedings of the 23rd ACM SIGKDD International Conference on Knowledge Discovery and Data Mining (pp. 1407–1416). ACM.
- Abernethy, J., & Hazan, E. (2016). Faster Convex Optimization: Simulated Annealing with an Efficient Universal Barrier. In International Conference on Machine Learning (pp. 2520–2528).
- Abernethy, J., Lahaie, S., & Telgarsky, M. (2016). Rate of Price Discovery in Iterative Combinatorial Auctions. In Proceedings of the 2016 ACM Conference on Economics and Computation (pp. 809–809). ACM.
- Azab, M., Mihalcea, R., & Abernethy, J. (2016). Analysing RateMyProfessors Evaluations Across Institutions, Disciplines, and Cultures: The Tell-Tale Signs of a Good Professor. In International Conference on Social Informatics (pp. 438–453). Springer International Publishing.
- Li, J., Ozog, P., Abernethy, J., Eustice, R. M., & Johnson-Roberson, M. (2016). Utilizing high-dimensional features for real-time robotic applications: Reducing the curse of dimensionality for recursive Bayesian estimation. In Intelligent Robots and Systems (IROS), 2016 IEEE/RSJ International Conference on (pp. 1230–1237). IEEE.
- Abernethy, J. D., Amin, K., & Zhu, R. (2016). Threshold bandits, with and without censored feedback. In Advances in Neural Information Processing Systems (To Appear) (pp. 4889–4897).
- Abernethy, J., & Johnson-Roberson, M. (2015). Financialized methods for market-based multi-sensor fusion. In IEEE/RSJ International Conference on Intelligent Robots and Systems.
- Abernethy, J., Lahaie, S., & Telgarsky, M. (2015). Price Discovery in Subgradient Combinatorial Auctions. In Auctions, Market Mechanisms, and Their Applications. AMMA Non-archival Track.
- Waggoner, B., Frongillo, R., & Abernethy, J. (2015). A Market Framework for Eliciting Private Data. In Advances in Neural Information Processing Systems 28.
- Abernethy, J., Lee, C., & Tewari, A. (2015). Fighting Bandits with a New Kind of Smoothness. In Advances in Neural Information Processing Systems 28.
- Abernethy, J., Chen, Y., Ho, C.-J., & Waggoner, B. (2015). Low-cost learning via active data procurement. In Proceedings of the Sixteenth ACM Conference on Economics and Computation (pp. 619–636). ACM.
- Abernethy, J., Kutty, S., Lahaie, S., & Sami, R. (2014). Information aggregation in exponential family markets. In (EC) Proceedings of the 15th ACM conference on Economics and computation (pp. 395–412). ACM.
- Wang, Q., Sheng, S.-P., Abernethy, J., & Liu, M. (2014). Jamming Defense Against a Resource-Replenishing Adversary in Multi-channel Wireless Systems. In (WiOpt) International Symposium on Modeling and Optimization in Mobile, Ad Hoc and Wireless Networks.
- Abernethy, J. D., Frongillo, R. M., Li, X., & Vaughan, J. W. (2014). A general volume-parameterized market making framework. In (EC) Proceedings of the 15th ACM conference on Economics and computation (pp. 413–430). ACM.
- Abernethy, J., Lee, C., Sinha, A., & Tewari, A. (2014). Online Linear Optimization via Smoothing. In (COLT) Conference on Learning Theory.
- Abernethy, J., Amin, K., Draief, M., & Kearns, M. (2013). Large-Scale Bandit Problems and {KWIK} Learning. In (ICML) Proceedings of the 30th International Conference on Machine Learning (pp. 588–596).
- McMahan, B., & Abernethy, J. (2013). Minimax optimal algorithms for unconstrained linear optimization. In (NIPS) Advances in Neural Information Processing Systems (pp. 2724–2732).
- Abernethy, J., & Kale, S. (2013). Adaptive Market Making via Online Learning. In (NIPS) Advances in Neural Information Processing Systems (pp. 2058–2066).
- Abernethy, J., Bartlett, P., Frongillo, R., & Wibisono, A. (2013). How to Hedge an Option Against an Adversary: Black-Scholes Pricing is Minimax Optimal. In (NIPS) Advances in Neural Information Processing Systems (pp. 2346–2354).
- Abernethy, J., Frongillo, R. M., & Wibisono, A. (2012). Minimax option pricing meets black-scholes in the limit. In (STOC) Proceedings of the 44th Symposium on Theory of Computing (pp. 1029–1040). ACM.
- Abernethy, J. D., & Frongillo, R. M. (2012). A Characterization of Scoring Rules for Linear Properties. In (COLT) Conference on Learning Theory.
- Abernethy, J., & Frongillo, R. M. (2011). A Collaborative Mechanism for Crowdsourcing Prediction Problems. In (NIPS) Advances in Neural Information Processing Systems (pp. 2600–2608).
- Abernethy, J., Chen, Y., & Wortman Vaughan, J. (2011). An optimization-based framework for automated market-making. In (EC) Proceedings of the 12th ACM conference on Electronic commerce (pp. 297–306). ACM.
- Abernethy, J., Bartlett, P. L., & Hazan, E. (2011). Blackwell approachability and low-regret learning are equivalent. In (COLT) Conference on Learning Theory.
- Abernethy, J., & Warmuth, M. K. (2010). Repeated Games against Budgeted Adversaries. In (NIPS) Advances in Neural Information Processing Systems (pp. 1–9).
- Abernethy, J., Bartlett, P. L., Buchbinder, N., & Stanton, I. (2010). A regularization approach to metrical task systems. In (ALT) Algorithmic Learning Theory (pp. 270–284). Springer Berlin Heidelberg.
- Abernethy, J., & Rakhlin, A. (2009). Beating the adaptive bandit with high probability. In (COLT) Conference on Learning Theory.
- Abernethy, J., Agarwal, A., Bartlett, P. L., & Rakhlin, A. (2009). A stochastic view of optimal regret through minimax duality. In (COLT) Conference on Learning Theory.
- Abernethy, J., Chapelle, O., & Castillo, C. (2008). Web spam identification through content and hyperlinks. In (AirWeb) Proceedings of the 4th international workshop on Adversarial information retrieval on the web (pp. 41–44). ACM.
- Abernethy, J., Bartlett, P. L., Rakhlin, A., & Tewari, A. (2008). Optimal strategies and minimax lower bounds for online convex games. In (COLT) Conference on Learning Theory.
- Abernethy, J., Hazan, E., & Rakhlin, A. (2008). Competing in the Dark: An Efficient Algorithm for Bandit Linear Optimization. In (COLT) Conference on Learning Theory. PDF** COLT 2008 Best Student Paper Award & 2008 Pat Goldberg Memorial Best Paper Award from IBM **
- Abernethy, J., Warmuth, M. K., & Yellin, J. (2008). Optimal strategies from random walks. In (COLT) Conference on Learning Theory.
- Rakhlin, A., Abernethy, J., & Bartlett, P. L. (2007). Online discovery of similarity mappings. In (ICML) Proceedings of the 24th international conference on Machine learning (pp. 767–774). ACM.
- Abernethy, J., Bartlett, P., & Rakhlin, A. (2007). Multitask learning with expert advice. In (COLT) Conference on Learning Theory.
- Abernethy, J., Langford, J., & Warmuth, M. K. (2006). Continuous experts and the binning algorithm. In (COLT) Conference on Learning Theory.
Technical Report
- Waggoner, B., Frongillo, R. M., & Abernethy, J. D. (2017). Addendum to "A Market Framework for Eliciting Private Data". CoRR (Vol. abs/1703.00899). Retrieved from http://arxiv.org/abs/1703.00899
- Kodali, N., Abernethy, J. D., Hays, J., & Kira, Z. (2017). How to Train Your DRAGAN. CoRR (Vol. abs/1705.07215). Retrieved from http://arxiv.org/abs/1705.07215
- Abernethy, J. D., Anderson, C., Dai, C., Farahi, A., Nguyen, L., Rauh, A., … Yang, S. (2016). Flint Water Crisis: Data-Driven Risk Assessment Via Residential Water Testing. CoRR (Vol. abs/1610.00580). Retrieved from http://arxiv.org/abs/1610.00580
- Abernethy, J., & Hazan, E. (2015). Faster Convex Optimization: Simulated Annealing with an Efficient Universal Barrier. CoRR (Vol. abs/1507.02528). Retrieved from http://arxiv.org/abs/1507.02528
- Abernethy, J. D., Lee, C., & Tewari, A. (2015). Spectral Smoothing via Random Matrix Perturbations. CoRR (Vol. abs/1507.03032). Retrieved from http://arxiv.org/abs/1507.03032
- Abernethy, J. D., Chen, Y., Ho, C.-J., & Waggoner, B. (2015). Actively Purchasing Data for Learning. CoRR (Vol. abs/1502.05774). Retrieved from http://arxiv.org/abs/1502.05774
- Abernethy, J., Kutty, S., Lahaie, S., & Sami, R. (2014). Information Aggregation in Exponential Family Markets. CoRR (Vol. abs/1402.5458).
- Abernethy, J., Lee, C., Sinha, A., & Tewari, A. (2014). Online Linear Optimization via Smoothing. CoRR (Vol. abs/1405.6076).
- Abernethy, J., Frongillo, R. M., & Wibisono, A. (2012). Minimax Option Pricing Meets Black-Scholes in the Limit. CoRR (Vol. abs/1202.2585).
- Abernethy, J., & Frongillo, R. M. (2011). A Collaborative Mechanism for Crowdsourcing Prediction Problems. CoRR (Vol. abs/1111.2664).
- Abernethy, J., Chen, Y., & Vaughan, J. W. (2010). An Optimization-Based Framework for Automated Market-Making. CoRR (Vol. abs/1011.1941).
- Abernethy, J., Bartlett, P. L., & Hazan, E. (2010). Blackwell Approachability and Low-Regret Learning are Equivalent. CoRR (Vol. abs/1011.1936).
- Abernethy, J., Agarwal, A., Bartlett, P. L., & Rakhlin, A. (2009). A Stochastic View of Optimal Regret through Minimax Duality. CoRR (Vol. abs/0903.5328).
- Bach, F., Abernethy, J., Vert, J.-P., & Evgeniou, T. (2008). A New Approach to Collaborative Filtering: Operator Estimation with Spectral Regularization. CoRR (Vol. abs/0802.1430).
- Abernethy, J., Bach, F., Evgeniou, T., & Vert, J.-P. (2006). Low-rank matrix factorization with attributes. CoRR (Vol. abs/cs/0611124).
Open Problems
- Abernethy, J., & Mannor, S. (2011). Does an Efficient Calibrated Forecasting Strategy Exist? (COLT) Conference on Learning Theory.
- Abernethy, J. (2010). Can We Learn to Gamble Efficiently? (COLT) Conference on Learning Theory.
- Abernethy, J., & Rakhlin, A. (2009). An Efficient Bandit Algorithm for root-T-Regret in Online Multiclass Prediction? (COLT) Conference on Learning Theory.
- Abernethy, J., & Warmuth, M. K. (2009). Minimax Games with Bandits. (COLT) Conference on Learning Theory.
Talks
Building Algorithms by Playing Games
Deviation Inequalities in Machine Learning
Online Learning, Regret, and Finance
Learning, Optimization, and the Benefits of Noise
Price Discovery in Subgradient Combinatorial Auctions
Data Science and the Flint Water Crisis
Faster Convex Optimization: Simulated Annealing with an Efficient Universal Barrier
Minimax Option Pricing Meets Black-Scholes in the Limit
Prediction, Belief, and Markets
A Collaborative Mechanism for Crowdsourcing Prediction Problems
Blackwell Approachability and No-Regret Learning are Equivalent
WITCH: A New Approach to Web Spam Detection
Fun Facts
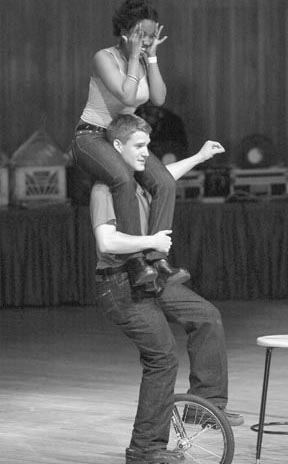
In a previous life, I used to do comedy juggling shows. I managed to win Funniest Student at UMass while studying there, and later got the chance to open for the comedian Sinbad and later Dave Chappelle. The Daily Hampshire Gazette ran a flattering article about my show right before the Sinbad appearance.
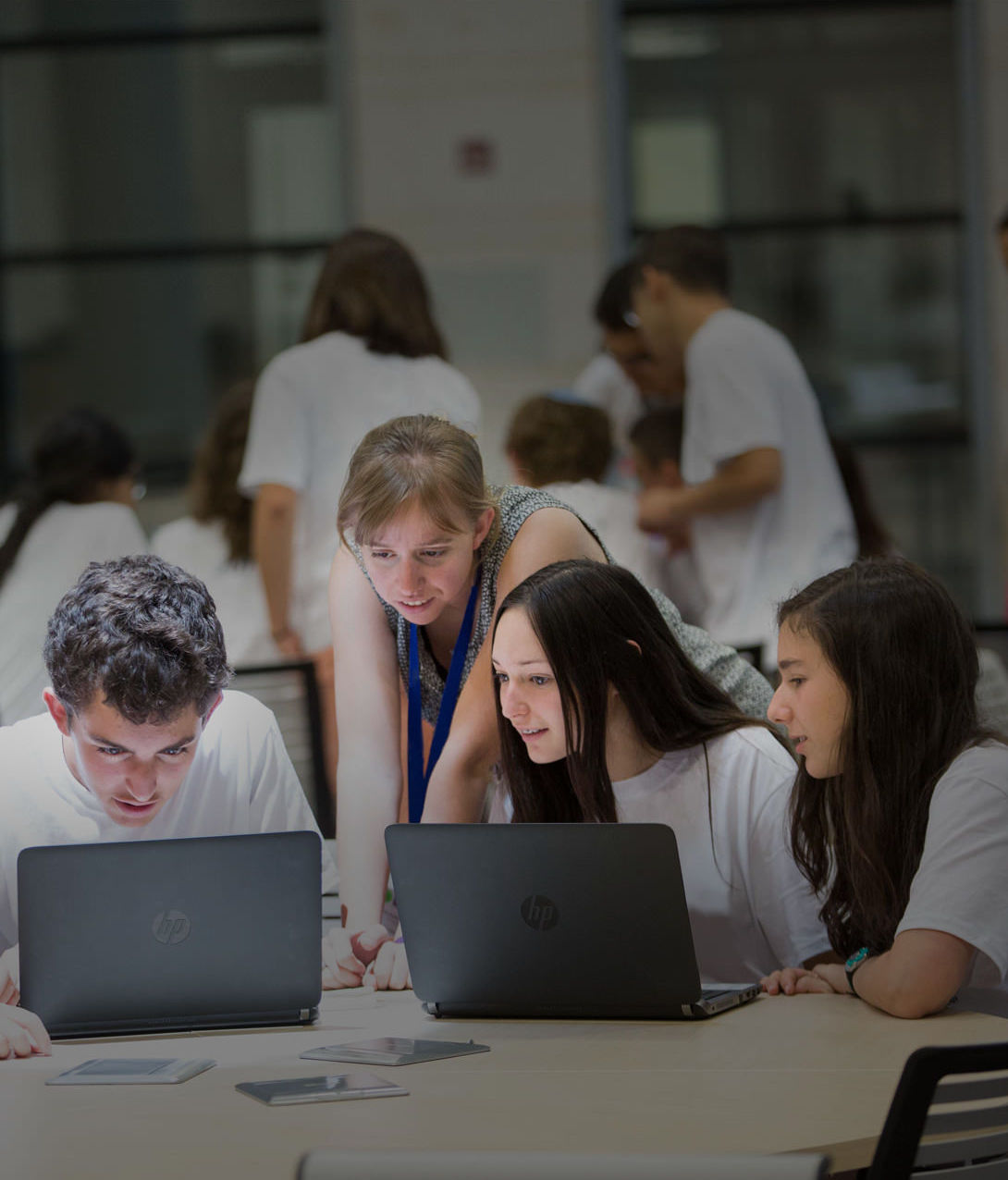
I used to help manage MEET: Middle East Education through Technology, an educational initiative aimed at bringing together Israeli and Palestinian youth. This project has brought me to Jerusalem three times.
I love playing Racquetball.
I have a cool ass sister who built this website for me
I rode a bicycle from Boston to San Francisco during the summer of 2002 with two friends, Keith Vanderlinde and Damien Burke.
My mom, an expert on Anthrax and Anthrax Vaccine, has a very popular informational Anthrax Vaccine Web site and blog.
Courses I have taught
Number | Name | Intitution | Year | |
---|---|---|---|---|
CS 7505 | Machine Learning Theory | Georgia Tech | Fall 2018 | VIEW |
CS 4540 | Advanced Algorithms for Machine Learning | Georgia Tech | Fall 2018 | VIEW |
CS 7505 | Machine Learning Theory | Georgia Tech | Fall 2017 | |
EECS 445 | Machine Learning | Univ. Michigan | Fall 2016 | VIEW |
EECS 545 | Graduate Machine Learning | Univ. Michigan | Winter 2016 | VIEW |
EECS 598 | Theoretical Foundations of Machine Learning | Univ. Michigan | Fall 2015 | VIEW |
EECS 203 | Introduction to Discrete Mathematics | Univ. Michigan | Fall 2014 | VIEW |
EECS 203 | Introduction to Discrete Mathematics | Univ. Michigan | Winter 2014 | VIEW |
EECS 598 | Prediction and Learning: It's Only a Game | Univ. Michigan | Fall 2013 | VIEW |